Predicting Checkpoint Inhibitor Immunotherapy Efficacy for Cancer Using Routine Blood Tests
A study has developed a method to predict the efficacy of checkpoint inhibitor immunotherapy in cancer patients using routine blood tests. The research involved analyzing data from thousands of patients across multiple cohorts, identifying key clinical and blood test features associated with treatment outcomes. The study's findings could help in personalizing cancer immunotherapy, improving patient outcomes by predicting who is most likely to benefit from these treatments.
The study protocol received approval from the institutional review boards at Icahn School of Medicine at Mount Sinai and MSKCC, with informed consent obtained from all participants.
A real-world cohort of 3,278 patients treated with at least one dose of immune checkpoint inhibitors (ICI) from 2014 through 2019 was analyzed. After exclusions, the cohort consisted of 2,035 patients across 17 cancer types, with a median age of 63.50 years and 57.20% being male. The most common cancer types were NSCLC, RCC, melanoma, H&N cancer, and bladder cancer.
Demographic and clinical data, along with 47 features from blood tests, were collected. After removing features with high missing values, 48 features from demographic, clinical, CMP, and CBC data were used for analysis. Tumors were staged following the American Joint Committee on Cancer guidelines.
Overall survival was calculated from the first ICI infusion to death, with clinical benefit classified based on RECIST v1.1 criteria. The study found that patients with stable disease for six months or more had similar overall survival outcomes to those with partial response.
Feature selection analyses identified 22 variables significantly associated with clinical benefit and 33 variables with overall survival. The study trained three different classifiers and three survival models to predict treatment outcomes, using a combination of classic and machine learning approaches.
Patients were stratified into high, moderate, and low-risk groups based on risk scores. The study found significant differences in clinical benefit rates and overall survival among these groups, supporting the model's predictive power.
The study compared the predictive power of the machine learning models with TMB and PD-L1 staining, finding that the models provided additional prognostic information beyond these biomarkers.
Using SHAP method, the study examined the impact of each feature on the model's predictions, providing insights into the biological mechanisms underlying treatment response.
RNA sequencing was performed on tumor samples to explore the relationship between gene expression and treatment outcomes, further validating the model's predictions.
This comprehensive analysis demonstrates the potential of routine blood tests and machine learning models to predict the efficacy of checkpoint inhibitor immunotherapy in cancer patients, offering a pathway towards more personalized and effective treatment strategies.
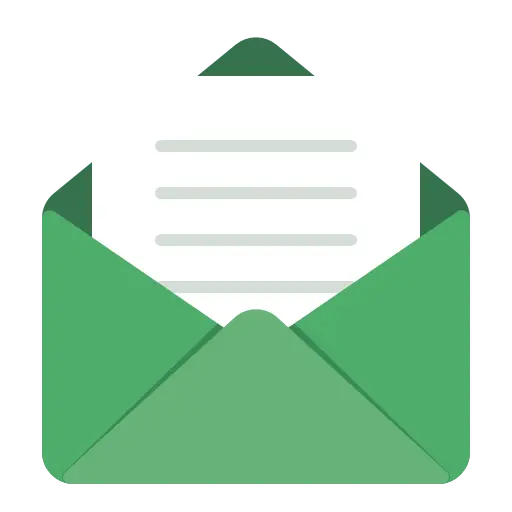
Stay Updated with Our Daily Newsletter
Get the latest pharmaceutical insights, research highlights, and industry updates delivered to your inbox every day.
Related Topics
Reference News
[1]
Prediction of checkpoint inhibitor immunotherapy efficacy for cancer using routine blood ...
nature.com · Jan 6, 2025
The study protocol was approved by institutional review boards, with informed consent from all patients. A real-world co...